Random thoughts as I scan through the book:
Central framing: learning as a means to generalize + predict
Key Tasks
- regression (predict a value)
- binary classification (sort an example into a boolean class of Y/N)
- multi-class classification (sort an example into multiple classes)
- ranking (sorting an object into relevant order)
Optimality of Bayes Optimal Classifier
If you have the underlying data distribution, we can classify \(y\) from \(x\) by choosing:
\begin{equation} f(x) = \arg\max_{y \in Y} P(x, y) \end{equation}
where \(P(a,b)\) is the probability of \(a,b\) pain on the data \(D\); but like you don’t have the probability distributed over \(D\), so sadge.
Optimality:
assume for contradiction \(\exists\) some \(g\) which works better than \(f\), meaning \(\exists x: g(x) \neq f(x)\). The error rate of \(f\) on \(x\) is \(1-P(x, f(x))\), of \(g\) is \(1-P(x, g(x))\). Yet, \(P(x,f(x))\) is maximized by definition of \(f\), so \(1-P(x, f(x)) \leq 1-P(x,g(x))\), meaning, \(P(x,g(x)) \leq P(x,f(x))\); recall \(P\) is the distribution on actual data so \(g\) is worse than \(f\), reaching contradiction. \(\blacksquare\)
Decision Tree
Its usually presented in terms of Gini impurity in a multi-class setting; as in the “score” is formalized as “Gini Purity”:
\begin{equation} G_{f} = \sum_{j=1}^{N} p_{f}(j) \cdot p_{f}(\neg j) \end{equation}
with \(N\) classes and \(f \in F\) features, and where \(p_{f}(j)\) means the probability that, for the subset of the data with condition \(f\), a random sample takes on class \(j\). Then, we select the feature:
\begin{equation} f_{split} = \arg\min_{f}\qty( G_{f} + G_{\neg f}) \end{equation}
We want this because we want each feature split to be “pure”—we want there to be either high probability of each split containing one class, or absolutely not containing it, so either \(p_{f}(j)\) or \(p_{f}(\neg j)\) should be very low.
Yet, this textbook uses a goofy representation where their splitting score is, after splitting by some feature \(f\) the count in each group having the majority class of that group—which functionally measures the same thing (class “purity” of each group); ideally, we want this value to be high, so we take argmax of it.
also I’m pretty sure we can recycle features instead of popping them out; check me on this.
Bias
Inductive Bias
Inductive Bias is defined here as the bias towards a particular choice of solution in a set of possible variations of valid solutions in absence of data which further narrows down the relevant concept.
Nice.
Confused about their treatment of parity, will come back later.
So true
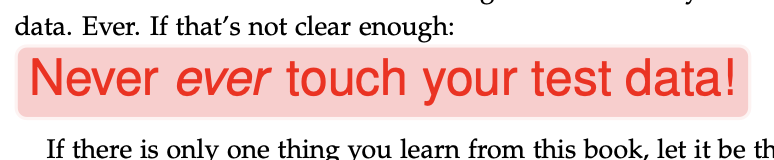
so true
Sooo true
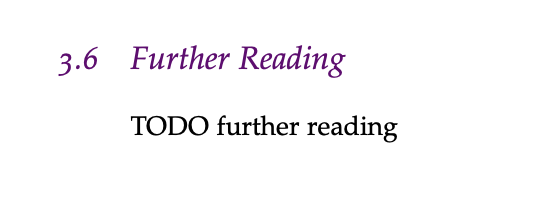
I officially have no opinions on their treatment of KNN/kmeans or single layer perceptrons
I wish there was a proof for why kmeans works
Perception
See logistic regression and Neural Networks
Perception Convergence
Suppose \(D\) is linearly separable with margin \(\gamma >0\) (otherwise it wouldn’t be very separable); assume \(\mid x \mid \leq 1\); then, 1-layer perceptrons converge in \(\frac{1}{\gamma^{2}}\) updates.
Sketch: take the fact that \(w^{(k)} = w^{(k-1)} + yx\). Compare it to \(w^{*}\) to obtain that \(w^{*} \cdot w^{(k)} \geq k \gamma\). Norm it and because \(x\) is within \(1\) so \(\mid w^{(k)}\mid^{2} \leq k\). Now do algebra.
We will obtain \(k \leq \frac{1}{\gamma^{2}}\).